SMA Collection Proclivity Predictor – Indian Bank
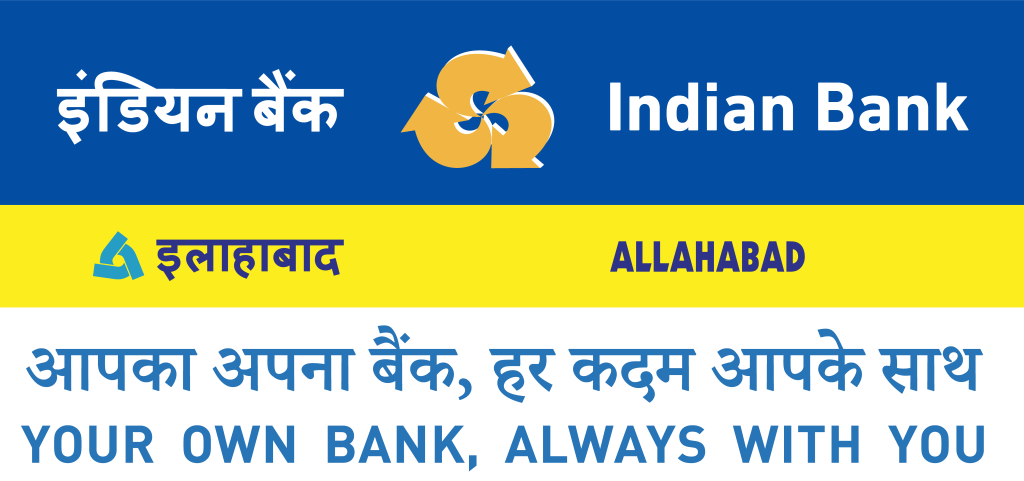
Proactive Risk Management for Early SMA Detection
Problem
- Difficulty in detecting early risks in SMA accounts, making it harder for banks to take preventive actions.
- Lack of effective strategies for preventing NPAs, affecting the overall financial health of banks.
- Challenge in improving asset quality by differentiating between high-risk and low-risk accounts.
- Absence of a clear, actionable plan for field functionaries to prevent SMA accounts from turning into NPAs.
- Difficulty in delivering actionable insights to field functionaries to enhance their effectiveness in risk management.
Solution
- Develop a machine learning model to identify high-risk SMA accounts early, enabling proactive risk management.
- Use a supervised learning approach to classify NPA and non-NPA accounts based on key variables.
- Create risk categories to improve model accuracy and targeting.
- Incorporate demographic, customer-level, account-level, and transaction data for comprehensive risk analysis.
- Preprocess and standardize data to ensure accurate model training and reliable predictions.
Outcomes
- Prevented Rs. 1597.54 Crores in potential slippage from high-risk accounts during the evaluation period.
- Reduced slippages by focusing on high-risk accounts and implementing data-driven actions.
- Minimized financial risks associated with NPAs by proactively addressing potential defaults.
- Reduced time and effort in collections, lowering operational costs and increasing productivity without additional resources.
- Improved operational efficiency by providing a prioritized collection journey for field agents and call center staff.
Challenges
- Data integration challenges due to missing, inconsistent, or inaccurate data, requiring extensive preprocessing.
- Model complexity from developing separate models for different loan types, with ongoing accuracy tuning.
- Difficulty in bringing model outputs to field functionaries before last-mile integration.
- Operational challenges in scaling the model across regions with varying conditions.
- Balancing precision and recall to minimize false positives and negatives required careful adjustments.
Innovation
- Tailored models for different loan categories to improve prediction accuracy.
- Integration of prescriptive actions to support better decision-making.
- Early stakeholder feedback ensured the model met business needs.
- Last-mile integration boosted adoption among field functionaries.
- Ongoing collaboration ensured a user-friendly and effective solution.
SKOCH Award Nominee
Category: Corporate Excellence
Sub-Category: Technology Development
Project: SMA Collection Proclivity Predictor
Start Date: 2-01-2023
Organisation: Indian Bank
Respondent: Himanshu Kansal
https://www.indianbank.in/
Level: BFSI – 4
Video
See Presentation
Gallery
Case Study
Proactive Risk Management for Early SMA Detection
Introduction
This project focuses on early risk detection in Special Mention Accounts (SMA) using a predictive model built by the Analytics Centre of Excellence. Tailored for various loan types, it enables proactive intervention to prevent NPAs, enhances asset quality, and integrates seamlessly with field applications to support timely, data-driven decisions.
Problems
The bank faced several challenges in managing high-risk Special Mention Accounts (SMA) that could potentially turn into Non-Performing Assets (NPAs). Early detection of these accounts was difficult, hindering proactive risk management. Without timely identification, SMA accounts were slipping into NPAs, negatively impacting the bank’s financial health. Additionally, there was a lack of clear differentiation between high-risk and low-risk accounts, making asset quality improvement challenging. Field functionaries also lacked an actionable plan to prevent SMA accounts from deteriorating. Furthermore, delivering actionable insights to field agents in a timely and effective manner was a persistent issue, leading to inefficiencies and delays in addressing potential risks.
Solutions
The bank developed a predictive,prescriprive and last mile integrated model using machine learning to identify high-risk SMA accounts early. This model leveraged data analytics, processing large amounts of data to predict which SMA accounts were most likely to become NPAs. A supervised learning approach was used, classifying accounts based on various independent variables. Separate models were developed for different loan categories (Retail, Corporate, MSME) to improve accuracy. The model included prescriptive actions in the form of customized collection journey that guided field staff to prevent SMA account becoming NPA. After internal testing and user acceptance testing, the model was integrated into mobile apps, call center portals, and dashboards for cohesive execution of prescriptive actions, ensuring seamless access for field functionaries to act on insights quickly.
Outcomes
The predictive model yielded several positive outcomes. By focusing on high-risk accounts and taking data-driven actions, slippages were reduced, improving asset quality
Slippage ratio for Q2 FY 25 has come down from 1.77% to 1.06% YOY, Whereas H1 FY 25 Slippage ratio has reduced from 1.67% to 1.28% on YOY basis
Corporate Sector has Collection Efficiency of 99% as on 30.09.2024
During H1 FY 2024-25, Percentage of Fresh NPAs of Retail and Agriculture Sector to Total Standard Advance is 0.22% and 0.50%
SMA 1+2 has decreased from 4.62% to 4.59% as on 30.09.2024 over 30.09.2023.
In Retail Sector, SMA (1+2) has reduced from 7.64% to 6.23% as on 30.09.2024 on YoY basis.
In Agri Sector, SMA (1+2) has reduced from 5.92% as on 30.09.2023 to 5.65% as on 30.09.2024 .
In MSME Sector, SMA (1+2) has decreased to 10.49 % as on 30.09.2024 from 11.50% as on 30.09.2023.
It also helped in maintaining the collection efficiency in the range of 95%.
Reduction in 40% of the efforts at Call Centre for following up for SMA recovery. Reduction of manpower efforts from 24 days to 15 days per cycle.
The integration of a prioritized collection journey also enhanced operational efficiency, allowing field staff and Call centre agents to focus on high-priority accounts for better risk management.
Challenges
Several challenges arose during the project’s implementation. Data integration proved difficult as the bank had to combine data from multiple sources, dealing with missing, inconsistent, or inaccurate data. This required extensive preprocessing to ensure the model’s predictions were reliable. Developing separate models for different loan types added complexity, requiring continuous tuning to balance accuracy and avoid overfitting. Another challenge was the adoption of the model by field functionaries. Before last-mile integration, getting the model’s outputs into the field and ensuring its practical use was difficult, as there was resistance to change. Scaling the model across different regions and adjusting it to diverse loan types and customer behaviors also presented operational challenges.
Innovation
The project’s innovation lies in the creation of tailored predictive models for different loan categories—Retail, Corporate, and MSME—improving accuracy by addressing the specific characteristics of each segment. The inclusion of prescriptive actions customized for each accounts within the model empowered field staff with a consistent framework to prevent SMA account becoming NPA. Additionally, last-mile integration with the mobile app , call center portal and intranet portal (for personal visit, sending SMS,calling,automatic notice generation) helped in improving the adoption rate among field functionaries. Early stakeholder involvement ensured that the model met the bank’s practical business needs and increased user acceptance. This combination of data-driven risk identification, tailored solutions, and seamless integration marked a significant innovation in the bank’s approach to credit risk management.
Opportunities
Future opportunities include expanding the model to cover SMA 0 and Fresh NPA accounts. This will enable a comprehensive, 360-degree approach to managing Stressed asset, enhancing early risk intervention and streamlining monitoring processes for improved asset quality control.
Summary
This project developed a predictive , prescriptive and last mile integrated model for early risk detection in high-risk SMA accounts, using tailored analytics and last-mile integration. By enabling proactive management, reducing slippages, and enhancing operational efficiency, the model strengthens asset quality and minimizes NPA risks, setting a foundation for comprehensive SMA monitoring.
For more information, please contact:
Himanshu Kansal at Himanshu.Kansal@indianbank.co.in
(The content on the page is provided by the Exhibitor)